Exploring Random Data Analysis and Measurement Techniques
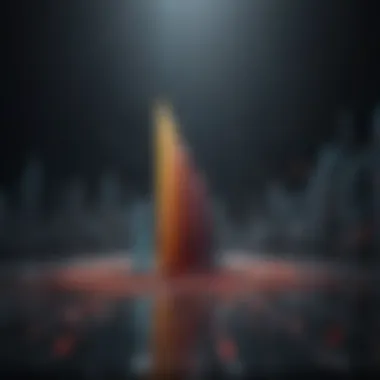
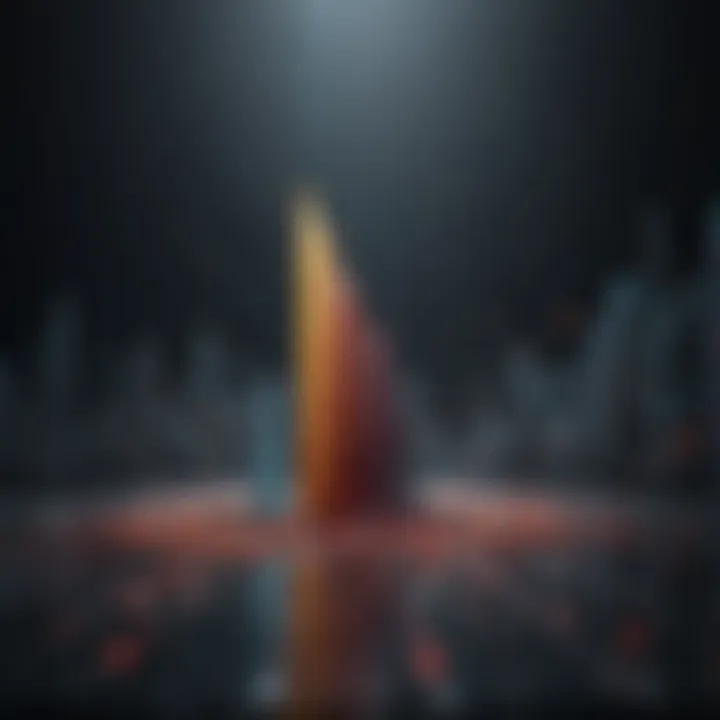
Intro
In an age characterized by an explosion of information, the ability to analyze and interpret random data has never been more crucial. Researchers in diverse fields—from social sciences to natural sciences—are increasingly depending on robust methodologies to decipher patterns from seemingly chaotic information. Understanding how to navigate the complex waters of random data analysis goes beyond mere calculation; it requires a keen insight into the underlying measurement procedures that govern data collection and interpretation.
Navigating this landscape, one must not only grasp the theoretical underpinnings but also the practical applications that these methods offer. This article endeavors to illuminate these essential aspects. Through a thorough exploration of key concepts, the article will provide clarity on the primary terms and relevant theories that shape this field, ultimately supporting scholars and practitioners in their pursuit of accurate and insightful research output.
Key Concepts
Definition of Primary Terms
To embark on this journey, one must first clarify what is meant by key terms in this domain:
- Random Data: Refers to data points collected in a manner that does not follow a predictable pattern. This randomness is fundamental to ensuring that the results can be generalized across larger populations.
- Data Analysis: The process of systematically applying statistical and logical techniques to describe and evaluate data.
- Measurement Procedures: The set of protocols and practices employed to gather data accurately. This includes defining variables and standardizing processes for consistency.
Understanding these terms lays the groundwork for deeper discussions about methodologies and practices in random data analysis.
Related Concepts and Theories
The backdrop of random data analysis is peppered with several crucial theories that enhance understanding:
- Statistical Theory: A branch of mathematics concerned with collecting, analyzing, interpreting, and presenting data. This often entails concepts like probability distributions and hypothesis testing.
- Inferential Statistics: A set of techniques that allow conclusions to extend beyond the immediate data. For instance, making predictions about a population based on a representative sample.
- Measurement Reliability and Validity: Key attributes for ensuring that data collected is both trustworthy and accurate. Reliability speaks to consistency across measurements, whereas validity measures how well a test actually assesses what it claims to measure.
Understanding these foundational concepts is critical for interpreting the complexities of random data and crafting effective measurement procedures.
Future Directions
Gaps Identified in Current Research
Despite the substantial body of work in the realm of random data analysis, some gaps remain:
- Integration of New Technologies: Many studies have yet to incorporate modern tools and software that could enhance data analysis, such as machine learning algorithms or advanced data visualization platforms.
- Focus on Data Ethics: The implications of data collection practices and the ethical dimensions surrounding privacy concern are often inadequately addressed.
Suggestions for Further Studies
Moving forward, research could benefit from a focused approach toward:
- Longitudinal Studies: Conducting extended research periods to assess data trends over time, which could validate current findings or uncover new phenomena.
- Interdisciplinary Approaches: Merging insights from various fields—like psychology, sociology, and economics—could lead to richer analyses and innovative methodologies.
Understanding Random Data
Understanding random data is essential for both researchers and practitioners in the scientific community. The study of such data allows for deeper insights into phenomena that might otherwise remain obscured. At its core, random data encompasses the idea that not all data can follow a predictable pattern, making it crucial to recognize the characteristics and implications of randomness in data sets.
One key aspect of understanding random data lies in its ability to mirror real-world complexities. Take, for instance, how consumer behavior varies with changing economic conditions. If we think of buying habits as random data, we can gather insights that help shape marketing strategies. Analyzing data that seems chaotic at first can reveal trends and correlations that guide decision-making.
Moreover, acknowledging the variability inherent in random data not only enriches analytical discussions but also prompts researchers to adopt a more flexible approach in their methodologies. Data doesn’t simply fit into neat little boxes; it often dances around the edges, defying rigid classifications. This adaptability becomes vital in ensuring accuracy in measurement and analysis.
Definition and Characteristics
Random data is primarily defined by its unpredictability. Unlike structured data, which follows a set pattern or sequence, random data does not lend itself to straightforward forecasting. In practical terms, this means that each data point gathered can vary widely, sometimes influenced by innumerable external conditions.
Characteristics of random data include:
- Unpredictability: Each occurrence stands separate and might not relate logically to others.
- Variation: The values can diverge for varied reasons, whether influenced by human actions, environmental changes, or other unseen factors.
- Diversity: It often showcases a spread of outcomes, thereby providing a richer canvas for analysis.
Understanding these characteristics helps in devising appropriate analytical techniques. For example, statistical methods such as regression and correlation become instrumental in interpreting the behavior of random data.
Importance in Research
The importance of understanding random data in research cannot be overstated. Random sampling methods, for instance, enhance the legitimacy of research findings. When researchers use techniques such as simple random sampling, they minimize biases, allowing for a more truthful representation of the larger population.
Here are some points highlighting the significance of random data in research:
- Informed Decisions: Findings derived from random data analysis allow professionals to base decisions on facts rather than conjecture.
- Enhanced Reliability: Random data lends itself to statistically sound conclusions, which lead to robust research outcomes.
- Broader Implications: Insights derived from random data can translate to larger trends across various fields like social sciences, health sciences, and even engineering.
Research grounded in an understanding of random data essentially provides a solid footing for further inquiries. It challenges researchers to question assumptions and explore unexpected relationships, ultimately enriching the knowledge landscape.
Theoretical Foundations of Randomness
Understanding the theoretical foundations of randomness is a linchpin for grasping how random data analysis operates within various domains—especially in research. It serves as the backbone for many statistical methods and informs the way data is collected, analyzed, and interpreted. When researchers understand the principles of randomness, it illuminates why certain results occur and aids in drawing more valid conclusions.
There are several key elements and benefits to consider within this framework, particularly statistical randomness and probabilistic models. Let’s take a closer look.
Statistical Randomness
Statistical randomness refers to patterns of outcomes that lack predictability and can better reflect true variability in the population being studied. This concept is paramount in experiments and surveys as it ensures that every data point is equally likely to be selected, which enhances the reliability of findings.
Key characteristics of statistical randomness include:
- Unpredictability: In random samples, it’s tough to discern any meaningful patterns in the data. This unpredictability is vital in scientific studies, as it mitigates biases that could distort results.
- Uniform Distribution: For true randomness, each element within a dataset should have an equal chance of occurrence. Such consistency allows researchers to generalize their findings more effectively when studying large populations.
- Effect on Statistical Inference: Analysis techniques such as hypothesis testing or confidence intervals hinge on this concept. When samples are derived randomly, the inferences drawn reflect the population closely, keeping potential errors in check.
One must keep in mind that achieving true statistical randomness can often be tricky. Researchers might face hurdles in designing studies that eliminate all biases and external factors.
Probabilistic Models
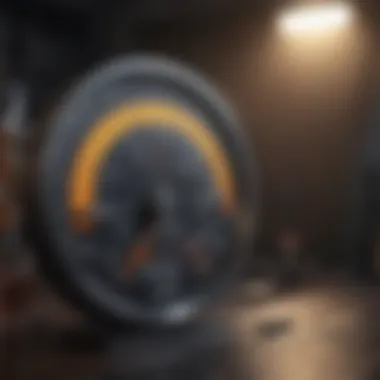
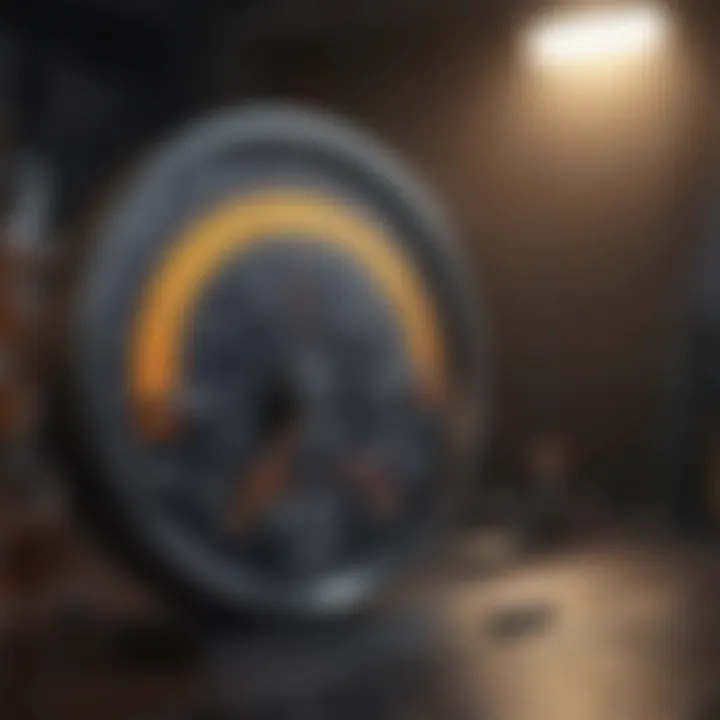
Probabilistic models sit at the heart of statistical theory, offering a structured way of analyzing random phenomena. These models provide a framework for making predictions and understanding the uncertainties inherent in sampling processes.
The significance of probabilistic models can be encapsulated in the following points:
- Predictive Power: These models allow researchers to forecast behavioral or event patterns based on historical data. For example, predicting the likelihood of a specific health outcome based on various demographic factors.
- Risk Assessment: In fields like finance and health sciences, probabilistic models help in assessing risks. They quantify uncertainties and aid in making informed decisions by illustrating various potential outcomes.
- Simplified Complexities: Real-world data often comes muddied with complexities. Probabilistic models break down these complexities into digestible parts, making it easier for researchers to comprehend intricate relationships.
By utilizing probabilistic models, researchers can navigate the chaotic nature of random data with a clearer lens, ultimately drawing more accurate and justified conclusions.
"The essence of probability is not in the sheer number of outcomes, but in understanding their stochastic nature to make informed decisions."
In summary, the theoretical foundations of randomness are crucial for the integrity of research methodologies. Whether through statistical randomness or probabilistic reasoning, these principles constitute the scaffolding that upholds rigorous analysis in data collection and interpretation.
Data Collection Techniques
Data collection techniques form the backbone of effective random data analysis. They encompass a myriad of methods aimed at gathering relevant information that can shape research findings and support inference-making. To understand the scope of these techniques is crucial because they not only affect the nature of the data collected but also the reliability and validity of the results derived from that data.
Choosing the right data collection method can likewise optimize the balance between time efficiency and data accuracy. Factors such as the specific research question, available resources, and the characteristics of the target demographic play pivotal roles in this decision-making process. Without a clear plan for data collection, one risks digging up results that lack real substance or, worse, are skewed by poor methodology. As such, the importance of establishing proper data collection techniques can never be overstated.
Surveys and Questionnaires
Surveys and questionnaires are among the most widely adopted techniques in research. This stems largely from their ability to cover a wide scope with relative ease and their capacity to reach numerous respondents quickly. For example, an online survey using tools like Google Forms can enable researchers to amass data from a diverse sample without the need for physical presence.
One of the key benefits of surveys is the ability to quantify sentiments, experiences, or behaviors. Researchers can structure questions in a manner that allows for both qualitative and quantitative data collection. However, a challenge that often crops up is question bias. Poorly worded questions may lead to misunderstandings, creating a ripple effect of inaccuracies in the data gathered. Therefore, a thorough pre-test of the survey is highly recommended to refine questions and ensure clarity.
Experimental Methods
Experimental methods go a step further by establishing a direct link between cause and effect. This is particularly beneficial in trials where researchers manipulate variables to observe outcomes. Classic examples include clinical trials for new medications, where patient responses can hinge on varying dosages or treatment combinations. Here, random assignment is key, helping to eliminate confounding variables that may otherwise skew results.
Nevertheless, while experimental methods often yield robust datasets, they must be meticulously designed. Factors like sample size, selection criteria, and control measures should be taken into account. Additionally, ethical considerations come into play, especially when dealing with human subjects, further complicating data collection in experimental settings.
Observational Studies
Observational studies are a treasure trove for data collection, particularly in natural settings where researchers observe behaviors in real time without interference. In psychology, for instance, researchers may study child interactions on a playground to gather insights about social behavior. This technique offers unmatched ecological validity, as it reflects genuine actions and reactions.
On the downside, one of the major concerns is the potential for researcher bias. Since the observer's interpretation can impact the data, it’s essential to maintain objectivity and employ systematic observation guidelines. Furthermore, observational studies typically lead to non-inferential data, meaning that while one may discern patterns, establishing causation can become a challenging endeavor.
"Understanding how we collect data is just as important as analyzing it. A strong collection method lays the groundwork for reliable analysis."
Analyzing Random Data
Analyzing random data is a cornerstone in the realm of statistics and research methodologies. Understanding how to interpret randomness effectively enhances the precision of conclusions drawn from varying data sets. It allows researchers to uncover patterns that might otherwise remain hidden, provides a pathway to understanding relationships between variables, and supports sound decision-making that echoes across disciplines.
Such analysis isn't merely academic; it's crucial for developing practical solutions in real-world contexts. Addressing specific elements such as descriptive and inferential statistics offers insight into the strengths and pitfalls of our approaches. The reliability of these analyses heavily impacts the overall comprehension of the data set at hand, shaping the narrative researchers express through their findings.
> "Analyzing random data transforms noise into meaningful patterns."
Descriptive Statistics
Descriptive statistics serve as the foundation for summarizing and presenting raw data in a comprehensible manner. By utilizing measures such as the mean, median, and mode, they encapsulate the core characteristics of the data. As a shrewd choice for researchers, these statistics offer a snapshot of what the data reveals without overwhelming interpretations or complicated models.
Measures of Central Tendency
Measures of central tendency are at the heart of descriptive statistics. They calculate the center of a data distribution, allowing one to represent vast amounts of information neatly. The essential characteristic of this aspect is that it provides a summary value that reflects the general tendency of the data. By being simple and intuitive, it becomes a popular choice for researchers focusing on initial data understanding.
A unique feature of these measures, like the mean, is its sensitivity to outliers. While an outlier can skew the mean significantly, the median offers robustness since it merely considers the middle point in sorted data. Such advantages show why researchers often lean towards using multiple measures to gain a more rounded understanding of their data.
Measures of Variability
Measures of variability delve deeper into the dataset by focusing on how much the data points differ from each other. Such metrics, including range, variance, and standard deviation, are pivotal in assessing the distribution's consistency. A key characteristic of variability measures is that they highlight the spread of data, providing insights into the reliability and stability of data trends. Their importance is seen remarkably in fields like social sciences, where variability can indicate diverse responses or behaviors.
One unique benefit of these measures is they inform researchers about how representative the averages (central tendencies) are. For instance, a low standard deviation suggests that data points are clustered closely around the mean, enhancing the trustworthiness of the central measure. However, too much variability can draw attention away from the central trends, potentially complicating interpretations. Thus, alongside central tendency measures, they form a comprehensive analytical strategy.
Inferential Statistics
Inferential statistics allow researchers to make predictions or generalizations about a population based on sample data. This branch of statistics extends beyond mere observation by drawing conclusions that might not be directly evident, and it plays a pivotal role in hypothesis formulation.
Hypothesis Testing
Hypothesis testing is a fundamental aspect of inferential statistics where researchers test assumptions about a population. It contributes significantly to shaping scientific discourse, allowing for a structured method to validate or challenge assumptions. The central characteristic of hypothesis testing is its systematic nature, providing clarity in decision-making through statistical evidences instead of subjective judgments.
An essential feature of hypothesis testing is the p-value, which helps determine the significance of results. A low p-value can reject the null hypothesis, indicating that the observed effect is likely not due to chance. However, the risk of Type I and II errors underscores the complexity of this method and necessitates careful consideration while interpreting results.
Confidence Intervals
Confidence intervals offer another layer in inferential statistics, depicting the range in which a population parameter is expected to lie based on sample data. They contribute to a more nuanced understanding of variability and uncertainty around estimates. A key characteristic of confidence intervals is that they communicate not only an estimate but also the degree of precision around this estimate, making them extremely beneficial for thorough and responsible research.
Unique to confidence intervals is their ability to reflect researcher confidence, often expressed in percentages (e.g., 95% confidence), allowing for clear communication of uncertainty. While they provide invaluable insight into data reliability, the choice of level—like 90%, 95%, or 99%—can manipulate the width of the intervals, possibly leading to misinterpretation if not clearly conveyed. Understanding their advantages and implications is key in drawing responsible conclusions from random data.
Measurement Procedures
Measurement procedures form the backbone of any rigorous data analysis initiative. These procedures establish how data is gathered, analyzed, and interpreted, laying down the framework that underpins research findings. Their significance cannot be overstated, as effective measurement ensures that the data collected is both reliable and valid. Without solid measurement techniques, even the most sophisticated analyses can lead researchers astray, producing results that are misleading at best or entirely erroneous at worst.
When discussing measurement procedures, several facets come into play: the types of measurement utilized, and the concepts of reliability and validity. Each contributes uniquely to the robustness of research, impacting how conclusions are drawn and ultimately the quality of insights provided.
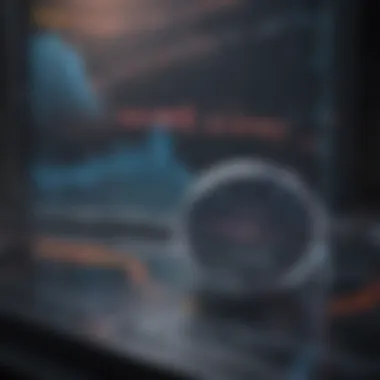
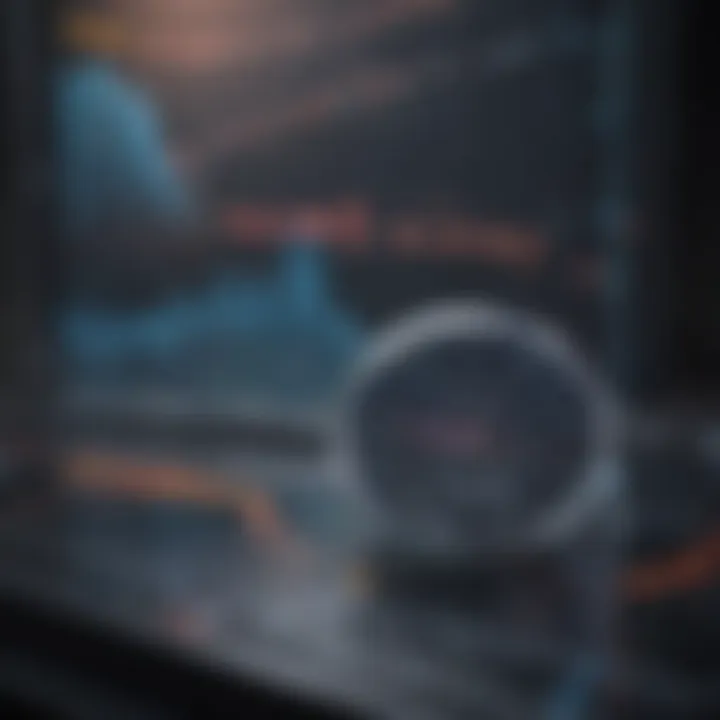
A sound understanding and implementation of measurement procedures pave the way for accurate and meaningful research outcomes.
Types of Measurement
Qualitative vs. Quantitative
Diving into the nuances of data collection, the distinction between qualitative and quantitative measurement is vital. Qualitative measures capture descriptive data — think interviews, open-ended surveys, or case studies that emphasize depth over breadth in understanding human experiences. Quantitative measures, on the other hand, focus on numerical data, often involving structured tools like closed-ended surveys or tests, allowing researchers to derive mathematical insights.
The primary characteristic for each is clear: qualitative deals with qualities and insights that numbers simply can't provide, while quantitative offers precision and the ability to statistically analyze trends. Qualitative methods can manifest rich narratives, providing context that numbers cannot explain; however, researchers might grapple with subjective interpretations. In contrast, quantitative approaches lend themselves well to statistical significance but might miss the subtleties of human experience. Each type bears unique advantages and disadvantages, clearly influencing the analysis direction and interpretation.
Nominal, Ordinal, Interval, and Ratio
When it comes to categorizing data, nominal, ordinal, interval, and ratio scales are fundamental. Nominal measurement classifies data into distinct categories without any order. Think of gender or eye color; there’s no inherent rank among them. Ordinal measurement, however, arranges data in a specific order but does not specify the difference between the ranks. For instance, ranking satisfaction on a scale of 1 to 5 conveys order but not consistent differences in satisfaction levels.
Interval scales provide insights about differences between values without a true zero point, such as temperature in Celsius. Finally, ratio scales embody the most comprehensive type of measurement; they include a true zero and allow for a full range of statistical analyses, like height or weight, making them incredibly beneficial for a plethora of studies. Each scale has its own strengths and weaknesses, shaping how data is interpreted and understood.
Reliability and Validity
In discussing measurement procedures, reliability and validity take center stage. Reliability refers to the consistency of a measure, indicating whether the same instrument yields similar results across different trials. High reliability means the measurement is trustworthy, but it doesn’t inherently signify accuracy; something could be consistently wrong. That's where understanding reliability comes in. It’s crucial for researchers wanting to ensure their studies are built on strong foundations. While reliability is beneficial for guaranteeing consistency in data, it is just the first half of the equation; without validity, even highly reliable measures might not reflect the reality of what's intended to be measured.
Assessing Validity
On the other hand, assessing validity addresses whether the measurement actually captures what it claims. It's essentially about the accuracy of the tools and methods employed, ensuring the data reflects true phenomena rather than artifacts of the measurement process. Validity encompasses various types, including content validity, construct validity, and criterion-related validity, each serving specific purposes. Understanding validity guarantees that the conclusions drawn from the data are sound and representative of the actual variables involved. While establishing validity can sometimes be more complex than reliability, its role is vital in ensuring that research findings are not only trustworthy but also relevant.
Common Challenges in Data Analysis
Data analysis is a pivotal aspect of research, especially when dealing with random data. Yet, it is not a walk in the park. Several hurdles can muddy the waters of clarity and accuracy. Understanding these challenges can significantly improve how researchers approach their data, ensuring more reliable outcomes. In this section, we will discuss some common complications that researchers encounter during data analysis. The challenges include bias in data collection, data overfitting, and incomplete or missing data, and each poses unique risks that can skew results.
Bias in Data Collection
Bias in data collection is like a pebble in your shoe; it's small but can cause significant discomfort. This type of bias occurs when the data collected does not represent the larger population accurately. For instance, if a survey predominantly reaches individuals from a particular age group, the findings may not serve as a reflection of the general populace. This scenario is often the result of poor sampling methods, which unfortunately can create a snowball effect, leading to distorted conclusions about a whole cohort.
To tackle this challenge, it’s essential to formulate a systematic approach:
- Random Sampling: Ensure that every member of the population has an equal chance of being selected. This method can help mitigate bias.
- Diversify Data Sources: Relying solely on one method can be limiting. Using various data collection methods can provide a broader perspective.
- Regular Reviews: Analyze the sampling methods regularly. This will help ensure they remain appropriate as the study progresses.
"In statistical analysis, not all data is created equal. Bias can mask the truth and lead to ruinous decisions."
Data Overfitting
Data overfitting is a common pitfall that researchers can fall into unknowingly. Picture it like trying to fit a square peg into a round hole; it's not going to work out in the long run. This occurs when a model is too complex, to the point where it starts capturing noise instead of the actual pattern. Basically, the model has tailored itself too closely to the training data, causing it to lose its predictive power when dealing with new, unseen data.
Here’s how to avoid overfitting:
- Simplify the Model: Use a simpler model that captures the essential patterns without getting bogged down by irrelevant data points.
- Cross-Validation: Implement cross-validation techniques to test how the model performs on different subsets of data.
- Regularization: Regularization techniques can help in penalizing overly complex models, ensuring the model focuses on the actual data patterns.
Incomplete or Missing Data
Incomplete or missing data is another head-scratcher for researchers. It's akin to attempting to complete a jigsaw puzzle with pieces missing; the picture just won't come together neatly. Missing data can arise from various sources, such as participant dropouts in studies or errors during data entry. Addressing this issue is crucial, as it can lead to biased estimates and diminished statistical power.
To handle incomplete data:
- Data Imputation: Consider using data imputation methods to estimate missing values based on available data.
- Sensitizing Analysis: Conduct sensitivity analyses to determine how missing data impacts the results and conclusions.
- Transparent Reporting: When publishing findings, be forthright about any missing data and how it was handled. Transparency is key to maintaining credibility and trust in research findings.
Mitigating these common challenges in data analysis doesn't guarantee perfection, but it certainly helps in navigating the stormy seas of random data analysis with more confidence and precision.
Statistical Techniques for Random Data
When we think about the world of statistics, we often find ourselves in a maze. Statistical techniques for random data are like the maps we need to navigate this maze. They guide researchers through the complexities of data, helping them to uncover insights that may otherwise remain hidden. In the realm of random data, these techniques play a pivotal role, as they assist in making sense of randomness and chance.
Using the right statistical techniques can illuminate patterns, guide decision-making, and support hypothesis validation. Researchers can identify correlations, assess variability, and ultimately derive meaningful conclusions from often chaotic datasets. Moreover, these methods allow for exploration beyond surface numbers, digging deeper into what the data is signaling.
Regression Analysis
Regression analysis is one of the cornerstones of statistical techniques employed for understanding relationships between variables. Think of it as trying to figure out how one thing affects another, much like how a robust tree bends with the wind—showing evidence of influence under external pressure. In regression, the goal is to model the relationship between a dependent variable and one or more independent variables.
For instance, consider a study analyzing how different levels of exercise affect weight loss. The dependent variable, weight loss, can be influenced by independent variables such as exercise duration, frequency, and type. By employing regression analysis, researchers can derive a mathematical model that predicts weight loss based on variations in exercise routines.
- Benefits of Regression Analysis:
- Predictive Analysis: It can anticipate trends and outcomes, a useful aspect for researchers and policymakers alike.
- Understanding Relationships: Illustrates how variables interact, helping to uncover hidden connections.
- Quantifying Effects: Helps in measuring the extent of influence one variable has on another.
However, regression analysis isn’t foolproof. It’s crucial for practitioners to remember that correlation does not imply causation. Universally applying regression findings without context might lead to incorrect conclusions.
ANOVA (Analysis of Variance)
ANOVA stands tall in the world of statistical techniques, providing a way to compare means across multiple groups. Rather than looking at two groups like in t-tests, ANOVA allows researchers to explore more extensive datasets in a single analysis. This can be likened to checking multiple roads for traffic conditions at once instead of just one.
Imagine a scenario where an educator wants to know if teaching methods impact student performance differently across several classes. By employing ANOVA, one can analyze test scores across multiple groups while controlling for other variables, ultimately determining whether any observed differences in scores are statistically significant.
- Key Points on ANOVA:
- Flexibility with Groups: Works well for various group sizes and is effective in balancing multiple comparisons.
- Identifying Variability: Helps in understanding not just the differences between group means, but how much variation exists within groups.
- Assumptions and Limitations: While ANOVA provides powerful insights, it is essential that the assumptions of normality and homogeneity of variance are met to trust the results fully.
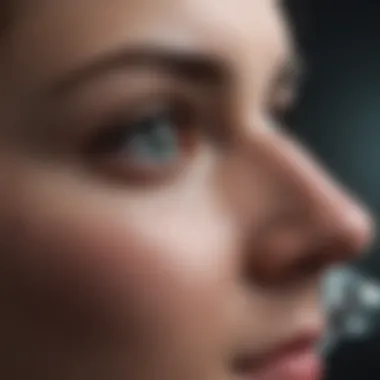
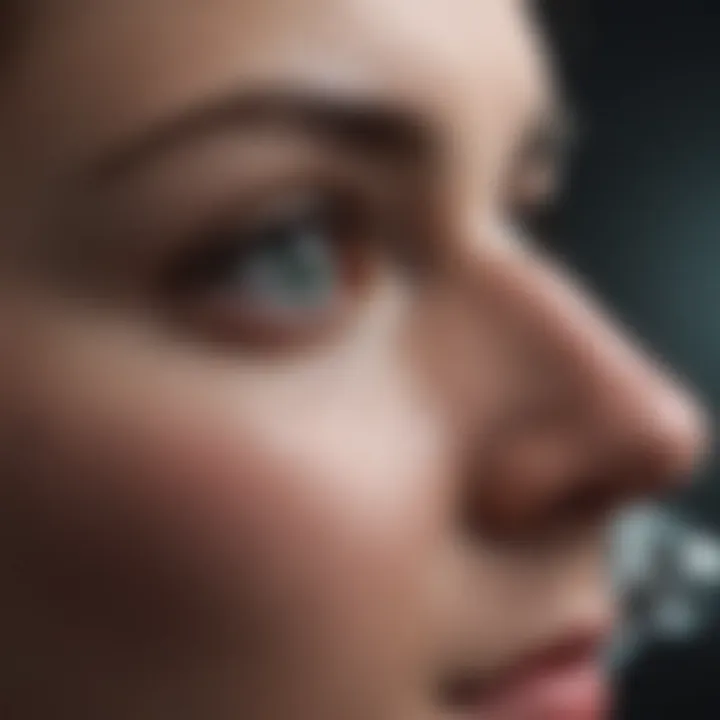
"Understanding the intricacies of ANOVA can save researchers from costly oversight in their analysis, paving the path to more authentic conclusions."
In summary, both regression analysis and ANOVA are vital statistical techniques for handling random data. They provide researchers with tools to dissect, understand, and interpret their findings with meticulousness. Adopting these techniques not only strengthens the analytical framework but also establishes a solid foundation for drawing credible conclusions in an uncertain world.
Interpreting Analysis Results
Interpreting analysis results is like piecing together a jigsaw puzzle, where each data point offers a crucial insight into the bigger picture. The ability to draw meaningful conclusions from random data isn't just a handy skill; it's a cornerstone of any research endeavor, be it in social sciences, health sectors, or engineering fields.
Understanding how to interpret results is vital because it equips researchers to engage thoughtfully with their findings. When results are interpreted clearly, they serve not only to validate hypotheses but also to illuminate underlying trends and relationships. This interpretation helps in making informed decisions and suggestions based on empirical evidence.
The benefits of honing this interpretative skill are substantial. A clear interpretation can:
- Offer clarity on the significance of the data
- Highlight correlations or patterns that could be pivotal
- Inform future research directions or policy decisions
Moreover, a meticulous approach can underscore the need for further inquiries. An unexamined result may mislead conclusions, potentially steering research in a wrong direction.
To achieve excellence in interpreting analysis results, it’s important to be aware of the various statistical metrics used and their implications. Understanding means evaluating context, recognizing variability, and discerning the data's nuances, which ultimately guides discussions or conclusions.
Drawing Closures
In drawing conclusions from analyzed data, one should tread carefully. It’s not merely about stating results; it's about synthesizing the information into a coherent narrative. In other words, what do the facts really say?
Here are some factors to consider when forming conclusions after analysis:
- Relevance of Findings: Ask if the findings directly address the research question. Understanding the context sets the stage for what follows.
- Causative Implications: Just because two variables are related doesn’t mean one causes the other. Critical thinking here is key.
- Statistical Significance: Is there a statistically significant relationship? Knowing the difference between correlation and causation is vital for sound conclusions.
Considering these elements leads not just to conclusions but to confidently articulated implications. Be clear and concise; conclusions should be accessible. They should enable readers to grasp the essence without getting mired in jargon or convoluted explanations.
Technical and Ethical Considerations
Delving into the technical and ethical aspects during result interpretation is like navigating through a minefield. It's essential to remain grounded in ethics while also ensuring that technical accuracy is upheld. Both aspects interact closely and shape how results are perceived and utilized.
- Transparency: Be clear about methods employed. Were the right tests conducted? This is crucial in ensuring reproducibility and trust in results.
- Data Integrity: Guard against manipulation or misinterpretation. Misleading representations can harm not just credibility but the larger scientific community's trust.
- Ethical Dilemmas: Consider the implications of how findings are presented and used. Misinterpretation can lead to poor policy decisions, affecting communities or individuals.
- Inclusivity: Acknowledge the limitations of your data and findings. Transparency in discussing flaws not only boosts validity but also reflects an ethical commitment to accurate representation.
"The greatest enemy of knowledge is not ignorance, it is the illusion of knowledge." - Stephen Hawking
As we move forward in understanding random data analysis, let us be those who are diligent, contemplating every figure, every data point, instead of hastily jumping to conclusions.
Applications of Random Data Analysis
Random data analysis has become a linchpin in various fields, providing insights that steer decisions and innovations. In the rapidly evolving landscape of research, employing random data analysis enables scholars and professionals to sift through noise and extract meaningful patterns. The ability to quantify uncertainty, ascertain trends, and dissect complex datasets is invaluable.
The tools and methodologies developed for analyzing random data are particularly important for establishing reliable conclusions. Researchers can make evidence-based decisions that resonate across disciplines. In essence, random data analysis opens the door to informed policy-making in sociology, medicine, and engineering, among others. Here’s how this plays out in different sectors.
Use in Social Sciences
The social sciences rely heavily on random data analysis to explore human behaviors, societal trends, and demographic patterns. Researchers routinely utilize surveys to gather data from diverse populations. Employing random sampling techniques ensures that the data collected reflects various viewpoints, allowing for a more comprehensive examination of social phenomena.
For instance, a study examining public opinion on a new policy might use randomized surveys. This way, every individual in the target population has an equal chance of being selected. The outcome is statistical findings that can influence public discourse and policy amendments. One notable example is the American National Election Studies, which utilize random sampling techniques to gauge voter behavior, providing crucial insights into electoral trends. This methodological rigor not only enhances the validity of research findings but also fosters trust within the broader community.
Applications in Health Sciences
In the domain of health sciences, random data analysis is crucial for clinical research and epidemiology. Randomized controlled trials (RCTs), often seen as the gold standard in medical research, harness the principles of randomness to ensure that the results are robust and generalizable. By randomly assigning participants to treatment or control groups, researchers can mitigate bias, thus providing clarity on the efficacy and safety of medical interventions.
Take, for example, a clinical trial investigating the effects of a new drug. Randomization helps keep the variables in check, thereby revealing precise effects of the drug without interference from confounding factors. Moreover, the analysis of large-scale health data can uncover patterns regarding disease prevalence and the impact of social determinants on health outcomes. This leads to targeted health policies and interventions that directly address identified needs in the community.
Implications for Engineering
Engineering too benefits greatly from random data analysis, where it serves as a cornerstone for quality assurance and predictive maintenance. Engineers employ statistical techniques to analyze data collected from sensors and machinery. By understanding failure probabilities and patterns, they can enhance designs, predict maintenance needs, and ultimately improve reliability.
For instance, in civil engineering, random sampling may be used to assess soil quality across a construction site, ensuring that the materials used meet safety standards. Furthermore, transient events, such as traffic fluctuations or electrical usage patterns, can be analyzed to optimize infrastructure and design better systems for energy distribution.
"The insights drawn from the randomness embedded in data not only guide decisions but also pave the way for innovation across various disciplines."
Overall, the applications of random data analysis bring precision to decision-making processes in social, health, and engineering domains. The analytical techniques not only enhance the validity of findings but also foster an environment of knowledge-driven strategies. Recognizing the importance of these applications is essential for both current and future research efforts.
The Future of Random Data Analysis
The landscape of random data analysis is evolving with rapid technological shifts and changing methodologies. This section delves into the prospects that lie ahead for researchers and practitioners in the field. As we step into an era marked by digital transformation and advanced computational capabilities, understanding this future is not just about adopting new tools; it’s about reshaping our approaches to analysis to enhance accuracy and insight.
Emerging Trends
Several trends are shaping the way we analyze random data. One of the foremost is the growing emphasis on big data analytics. With vast amounts of information flowing from diverse sources such as social media, IoT devices, and transactional records, the volume alone necessitates innovative analytical techniques. Traditional methods are often not sufficient to extract meaningful insights from this deluge.
Another observable trend is the rise of machine learning algorithms in data analysis. These algorithms can identify patterns that may go unnoticed with conventional statistical approaches. By learning from data, they refine their predictions and improve their accuracy over time. This shift towards machine learning doesn’t just automate processes; it enhances the scope and depth of analysis, leading to more nuanced conclusions.
Moreover, data visualization is taking on a more central role in the analysis process. As data becomes increasingly complex, the ability to present insights in an understandable and visually appealing manner is critical. Tools that offer interactive dashboards and real-time data monitoring are becoming essential in helping stakeholders make informed decisions quickly and efficiently.
"The future is not something we enter. The future is something we create." - Leonard I. Sweet
Technological Advancements
The next wave of advancements in random data analysis is undeniably tied to technology. The introduction of cloud computing is revolutionizing how data is stored and processed. With cloud services, researchers no longer need to invest in expensive physical infrastructures. They can access vast computing power on-demand, enabling them to analyze large datasets with relative ease. This accessibility opens the door for smaller organizations to engage in sophisticated analysis that was once out of reach.
Moreover, automation is playing a significant role in enhancing the accuracy and efficiency of data analysis. Tools that automate data collection, cleaning, and preprocessing minimize human intervention, thus reducing potential bias and error. Such automation not only streamlines the workflow but also allows analysts to dedicate more time to interpreting results and crafting actionable insights.
Finally, the integration of artificial intelligence into analytical procedures is taking center stage. AI-driven systems can recognize anomalies and highlight insights that human analysts might overlook. This capability is particularly important in fields like finance and healthcare, where understanding rare events can lead to critical strategic decisions.
In summary, the future of random data analysis promises a confluence of advancements that aim to enhance both the quality and efficiency of research. By embracing these trends and technological innovations, researchers and professionals can ensure their analytical practices remain robust and relevant in an ever-evolving landscape.