Integrating Ecology and Data Science for Environmental Insights
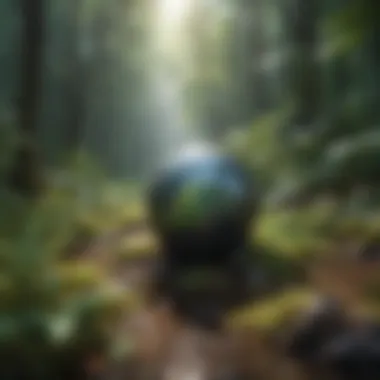
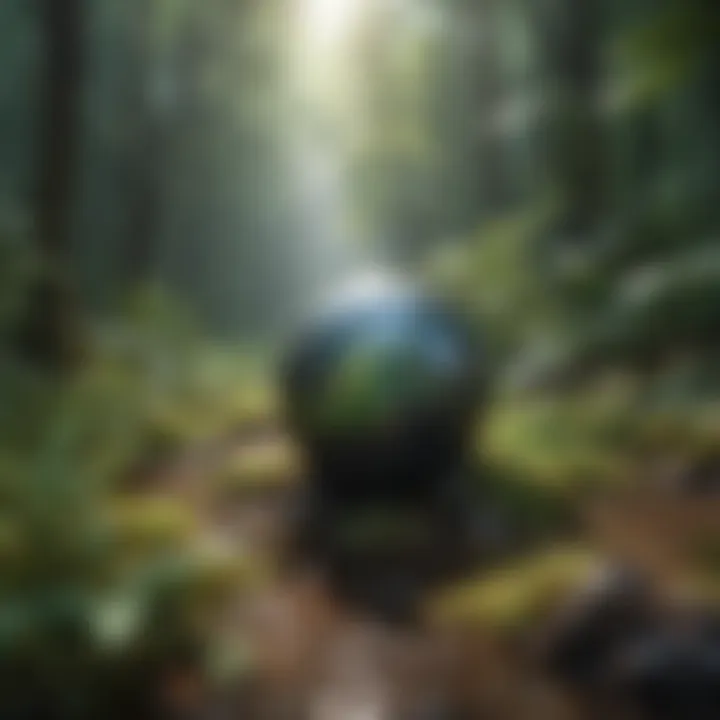
Intro
In today's world, where the environment is facing myriad challenges, the fusion of ecological principles with data science heralds a new dawn of understanding. The interlace of these two domains not only enhances scientific inquiry but also fosters a proactive approach to address pressing ecological issues. This exploration into ecological data science underscores the incredible potential of data analytics to unravel complex ecological patterns, enabling researchers and practitioners to make informed decisions based on solid evidence.
As we step deeper into this intricate landscape, we begin by unpacking the key concepts that form the foundation of ecological data science. Recognizing the relevance of each term and theory is vital for anyone aiming to navigate this fresh and exciting field. By shedding light on these fundamentals, we aim to equip our audience—students, researchers, educators, and professionals—with the knowledge and context needed to traverse this interdisciplinary terrain.
The narrative will unfold in a structured manner, first introducing essential terms and exploring related concepts that have shaped the practice of ecological data science. Following this, we will highlight existing gaps in current research and propose avenues for further exploration to ensure the continued evolution and impact of this discipline. Join us as we embark on this enlightening journey into the world where ecology meets data analysis.
Prolusion to Ecological Data Science
Ecological data science has emerged as a pivotal domain, marrying traditional ecological research with contemporary data analysis techniques. As we face monumental environmental challenges, understanding ecosystems profoundly and accurately is more essential than ever. This article aims to illuminate the importance of integrating data science into ecological studies, providing insights that not only help in conserving our natural world but also in enhancing our predictive capabilities regarding ecological phenomena.
The significance of ecological data science can be boiled down to a few key elements. First, it enables researchers to make sense of complex ecological data sets that often span vast geographical spaces and numerous variables. By employing statistical techniques, machine learning algorithms, and advanced data visualization methods, scientists can gain clarity on the intricate relationships within ecosystems.
Benefits of Ecological Data Science:
- Enhanced Decision-Making: With the aid of data visualization and modeling, stakeholders can make informed decisions concerning conservation efforts.
- Interdisciplinary Collaboration: Ecological data science fosters collaboration across various disciplines such as statistics, biology, and computer science. This synergy enhances the robustness of research outcomes.
- Rapid Responses to Environmental Changes: Thanks to real-time data analysis, researchers can quickly respond to changes in ecosystem dynamics, whether due to climate change, habitat loss, or pollution.
The considerations surrounding ecological data science are equally important. Researchers must ensure data quality and standardization to avoid misleading results. Techniques used in data management directly influence the integrity of research and the effectiveness of derived insights. Moreover, ethical considerations in data use must be highlighted continually. By understanding and complying with ethical standards, scientists can maintain public trust and ensure that their work benefits both ecosystems and communities alike.
“The future of ecological research lies in the hands of those who can artfully blend ecological insights with data-driven methodologies.”
In the upcoming sections, we will delve into what defines ecological data science, tracing its historical roots and evolution to appreciate its current state. This exploration will not only bring clarity to the concept but also set the stage for understanding the methodologies and applications that follow.
Core Components of Ecological Data Science
Understanding the core components of ecological data science is crucial to harnessing its potential for enhancing ecological studies. These foundational elements provide the structure upon which the analysis and interpretation of ecological phenomena stand. By focusing on frameworks, data collection techniques, and data management practices, we create a solid base that underpins the entire discipline. Each element plays a significant role in ensuring that the data collected is both relevant and reliable while also facilitating effective management of the insights derived from that data.
Ecological Frameworks
Ecological frameworks serve as a guiding structure for investigating the relationships between organisms and their environments. These frameworks draw upon principles from multiple ecological theories and disciplines, providing a comprehensive lens through which to observe ecosystems. The importance of these frameworks cannot be overstated, as they not only dictate the lines of inquiry but also influence the type of data collected and analyzed.
A well-defined framework allows researchers to analyze complex ecological interactions with clarity and precision. For example, the Landscape Ecology framework emphasizes the importance of spatial patterns and processes, aiding in understanding how ecological phenomena are influenced by spatial arrangements. This perspective can lead to better conservation strategies as it considers both temporal and spatial dynamics of ecosystems.
Moreover, frameworks often lead to the formulation of hypotheses that can be tested through data collection and analysis. They serve as the theoretical underpinnings that bridge the gap between ecological theory and practical data analysis.
Data Collection Techniques
The backbone of ecological data science hinges on the methods employed to gather data. Various data collection techniques must be tailored to the specific ecological inquiry. These techniques range from field surveys and remote sensing to automated sensor networks, each with its own advantages and challenges.
Field surveys, for instance, provide firsthand data on species distribution and abundance. They involve direct observation and interaction with the environment, enabling researchers to capture nuances that secondary data often miss. However, they can be resource-intensive, requiring significant time and labor.
On the other hand, remote sensing technologies like satellites and drones offer a broader perspective that can capture changes in landscape structures over time. The use of GIS can further enhance data richness by integrating geographic information with ecological data. This approach allows for layers of data analysis, making it easier to see patterns that might not be immediately apparent.
Regardless of the method used, researchers must maintain rigor in their data collection processes to ensure high-quality datasets. Accurate and standardized collection techniques are essential for reliable insights, which ultimately inform ecological models and conservation strategies.
Data Management Practices
Once data has been collected, the next critical step is its management. Effective data management practices not only facilitate organization but also ensure that data remains accessible and interpretable over time. This comes into sharper focus as researchers deal with vast amounts of diverse data.
Practices such as data standardization and documentation are paramount. Standardization allows for uniformity in the data, making it easier to compare across datasets. Meanwhile, thorough documentation provides context, making it possible for future researchers to understand and effectively utilize the findings.
Additionally, employing data management systems can greatly improve efficiency. Systems that allow for cloud storage and collaborative access can help researchers work together across locations and disciplines, fostering interdisciplinary communication and collaboration.
In summary, the core components of ecological data science, encompassing ecological frameworks, data collection techniques, and data management practices, form a comprehensive approach that shapes the future of ecological research. As we amplify our understanding of the natural world, it becomes increasingly apparent that investing time and resources into these areas is necessary for informing effective ecological policies and practices.
"The integration of ecological concepts with rigorous data practices not only enriches our understanding of nature but also enhances our ability to protect it effectively."
As the discipline evolves, a robust grasp of these foundational aspects will enable researchers to tackle the complex challenges that face our environments today.
Tools and Technologies in Ecological Data Science
In the realm of ecological data science, tools and technologies serve as the backbone, facilitating a deeper understanding of complex environmental systems. Such instruments are crucial not only for processing data but also for translating raw information into actionable insights. With the increasing amount of ecological data generated today, leveraging the right technologies is essential for researchers and professionals alike.
Statistical Software and Packages
Statistical software is undeniably a cornerstone in ecological analysis. Programs like R and Python offer a treasure trove of statistical packages tailored for ecological data. These tools enable researchers to conduct intricate analyses ranging from basic descriptive statistics to complex multivariate models.
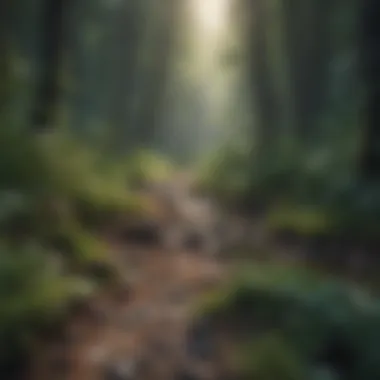
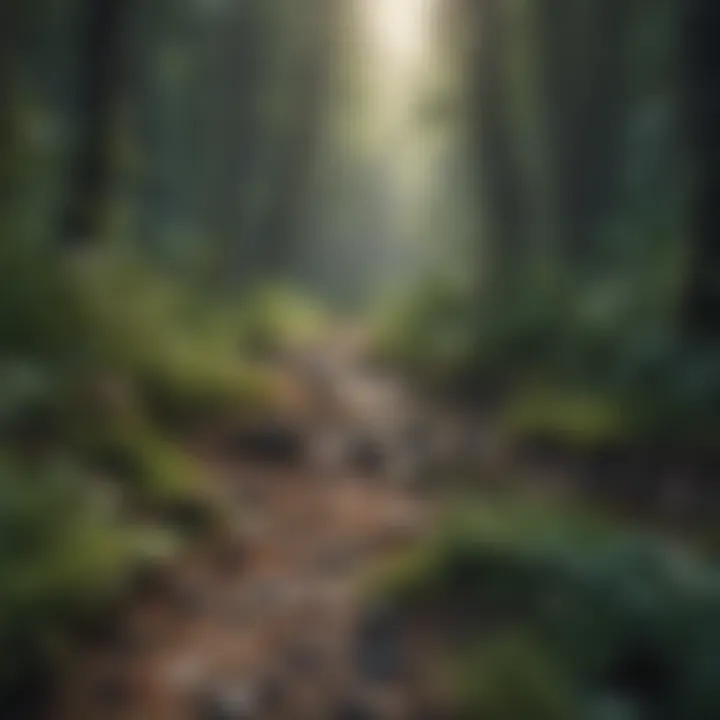
- R stands out due to its extensive libraries, such as vegan for community ecology or lme4 for mixed-effects models. Researchers can manipulate data and visualize results seamlessly, making it invaluable for field studies.
- Python, on the other hand, is gaining traction across various fields of data science. Libraries such as Pandas for data manipulation, and Matplotlib for visualization, allow ecologists to create sophisticated presentations of their findings.
Both R and Python encourage reproducibility and transparency, which are vital in ecological studies, allowing others to validate results easily.
"Statistical software not only handles the data but tells a story—transforming numbers into narratives that can influence policy and conservation efforts."
Geographic Information Systems (GIS)
GIS technology has transformed the way ecological research is conducted. Being able to map, analyze, and visualize spatial data brings a whole new dimension to understanding ecological phenomena. The integration of GIS into data analysis allows researchers to overlay various ecological factors—like species distribution, habitat spatial structures, and human development—on a map.
Some notable applications of GIS include:
- Habitat Suitability Modeling: By interpreting how various landscape features affect species presence, ecologists can identify regions where conservation efforts are most needed.
- Spatial Analysis: GIS can help visualize patterns of biodiversity, revealing hotspots that warrant protection or further research.
Moreover, the advent of mobile GIS applications enables fieldwork to be more efficient, allowing researchers to collect geodetic data on the go. This immediacy in data gathering strengthens ecological analysis by providing real-time insights into natural changes.
Remote Sensing Applications
Remote sensing technologies have revolutionized our ability to monitor and assess ecological environments on a large scale. The use of satellites and aerial sensors allows for the collection of data across vast and often inaccessible areas. This technological advancement plays a pivotal role in assessing landscape changes, vegetation health, and even animal movements.
Key uses of remote sensing in ecology include:
- Vegetation Monitoring: Satellites like Landsat track chlorophyll concentrations, providing vital clues to ecosystem health and productivity.
- Land Use Change Detection: Remote sensing can identify shifts from natural habitats to urban areas, offering insights critical for conservation planning.
Utilizing remote sensing data can lead to more informed decision-making in environmental management, ensuring that policies are backed by robust evidence and real-world scenarios.
As ecologists continue to integrate these tools and technologies, they enhance both the analysis and understanding of intricate ecological systems, ultimately paving the way for a more informed approach to conservation and resource management.
Methodological Approaches
In ecological data science, methodological approaches serve as the backbone of research, enabling scientists to analyze complex environmental systems. Employing various methodologies not only enhances data interpretation but also ensures that solutions to environmental problems are substantiated by robust evidence. This section discusses the different methodological frameworks that guide analysts in their quest to understand ecological patterns and trends, illustrating the importance of precision and rigor in methodology.
Modeling Techniques in Ecology
Modeling techniques are foundational in ecological data analysis, acting as lenses through which researchers can observe relationships within ecosystems. These models can represent the behavior of biological populations, the dynamics of ecosystems, or the interactions between different species. They offer ecological insights that sometimes cannot be observed directly in the field.
For example, population dynamics models help ecologists understand how species populations grow, shrink, or stabilize over time. By utilizing mathematical equations to simulate these dynamics, researchers can estimate future population sizes under various scenarios. This predictive capability is essential for conservation efforts, where anticipating changes can lead to more effective management strategies.
Additionally, habitat suitability models use a combination of field data and environmental variables to determine which habitats are best suited for particular species. They leverage statistical techniques to predict species distribution based on presence-absence data. Through these methods, conservationists can prioritize areas for protection and restoration.
Predictive Analytics and Machine Learning
Predictive analytics and machine learning represent a revolutionary shift in how we approach ecological research. These methods allow for the handling of vast data sets that occur in ecological studies, something traditional analytical methods often struggle with. By employing algorithms and advanced statistical techniques, scientists can uncover patterns and relationships in data that human analysis might overlook.
For instance, machine learning tools can process satellite imagery to monitor land use change over time or assess the health of ecosystems. These models learn from historical data and can predict future environmental changes, yielding far-reaching implications for urban planning and conservation strategies. Incorporating machine learning into ecological research can provide deeper insights into biodiversity assessments and extinction risk predictions. It automates complex data analysis tasks, increasing efficiency and accuracy.
Data Visualization Strategies
Data visualization is not just about pretty pictures; it plays a critical role in effective communication of complex ecological data. Through strategic visualization, researchers can distill intricate data into information that is accessible and meaningful to wider audiences, including policymakers, educators, and the general public.
Good visualization practices utilize a range of techniques, from heat maps to illustrate species distributions, to interactive dashboards that reveal real-time data insights. The goal is to tell a story—conveying messages that resonate and prompt informed decision-making.
Consider the use of geographic information systems (GIS), which combine maps with statistical analyses to visualize spatial patterns of ecological phenomena. By providing a layered view of data, GIS enhances understanding of how ecological factors interconnect, thus fostering better management and policy decisions.
"In a world overflowing with data, the ability to visualize it effectively is akin to possessing a compass that guides us through complex landscapes."
This commitment to methodological rigor ultimately shapes the decisions that affect our natural world.
Applications of Ecological Data Science
Applications of ecological data science hold considerable weight in modern environmental research and management. As the world grapples with challenges like habitat loss, climate change, and species extinction, understanding these applications provides a vital roadmap. Here, we’ll break down how ecological data science plays a pivotal role through three core uses: biodiversity assessment, climate change impact studies, and conservation planning and management.
Biodiversity Assessment
Biodiversity assessment is crucial to evaluating the health of ecosystems. Using ecological data science, researchers can analyze large sets of data from various sources—like satellite imagery, remote sensors, and field survey results—to categorize species and habitats effectively. Such assessments can reveal trends in biodiversity loss, helping to identify regions that need conservation efforts.
For example, by integrating machine learning algorithms with ecological data, scientists can predict the distribution of species based on historical ecological data and environmental variables. This predictive modeling is invaluable for conservation efforts, where knowing where a species is likely to thrive can lead to better-targeted actions.
Incorporating these assessments not only aids in compliance with global biodiversity targets, such as the Convention on Biological Diversity but also informs local stakeholders and policymakers about the intrinsic value of ecological resources in their region.
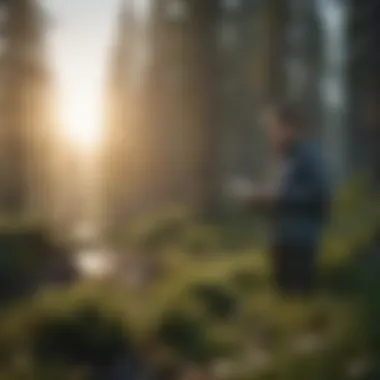
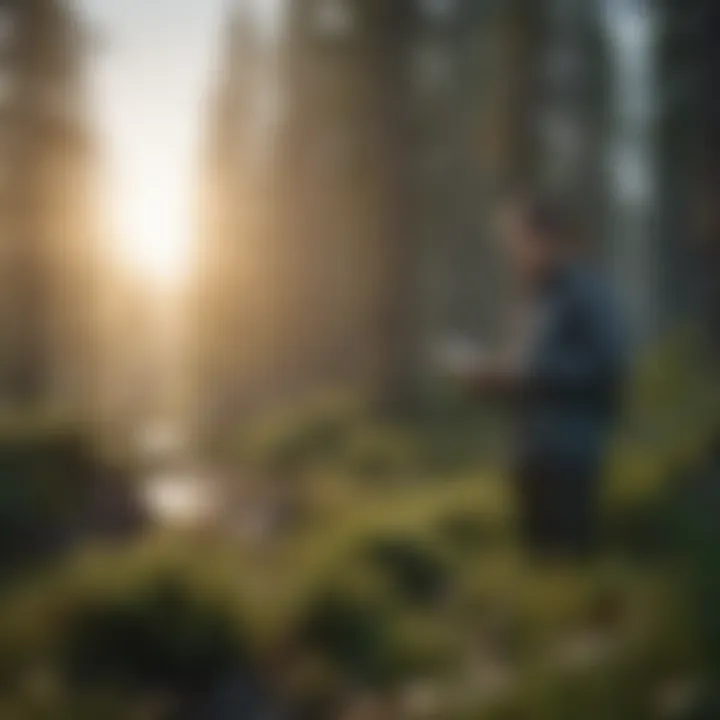
Climate Change Impact Studies
The impacts of climate change are far-reaching, and understanding these effects is paramount. Ecological data science enables the examination of how climate variables, such as temperature and precipitation patterns, affect ecosystems. Analyzing historical data alongside current trends allows researchers to draw connections between environmental changes and ecological responses.
For instance, one might discover that specific plant species are blooming earlier due to warmer temperatures, altering the food web for herbivores and their predators. With such insights, stakeholders can make informed decisions about mitigating these impacts.
Moreover, the use of extensive datasets, including climate models and ecological records, can lead to forecasting future scenarios. These projections are essential for developing adaptive management strategies to maintain ecosystem resilience, thereby ensuring stable natural resource availability.
Conservation Planning and Management
When it comes to conservation efforts, data-driven decision-making is critical. Ecological data science facilitates the coordination of conservation strategies by providing comprehensive insights into species populations, habitat integrity, and ecosystem services. By analyzing current trends and evaluating different management practices, practitioners can better allocate resources.
Take for example the case where predictive analytics are used to identify potential human-wildlife conflicts in a given area. By anticipating such interactions, conservation managers can establish proactive steps—like creating wildlife corridors to mitigate road fatalities.
Furthermore, public engagement is enhanced when local communities are presented with data-driven rationale behind conservation decisions. Leaders who communicate findings effectively can foster support and encourage participation, making conservation efforts more robust and sustainable.
"Data without a purpose is like a ship without a sail; it may float but not in the right direction."
In summary, the applications of ecological data science are pivotal in fostering a greater understanding of our fragile ecosystems. Through biodiversity assessments, climate change studies, and targeted conservation plans, data science offers a lens through which environmental challenges can be tackled effectively. As the field evolves, these applications will likely become essential tools for anyone looking to make a difference in ecological stewardship.
Challenges in Ecological Data Science
In the realm of ecological data science, recognizing the challenges is just as crucial as understanding its applications. Issues surrounding data quality, communication across various fields, and ethical considerations greatly affect the integrity and efficiency of ecological research. Tackling these challenges not only enhances the reliability of the data but also ensures that findings can effectively inform conservation policies and practices.
Data Quality and Standardization
When dealing with ecological data, the accuracy and consistency of the data play a vital role in the validity of research outcomes. Poor data quality can lead to misleading interpretations which ultimately affect the management of ecosystems. Standardizing data collection and analysis methods ensures comparability across studies. This is easier said than done, given the varied sources of data involved. For instance, biodiversity assessments may pull in data from field surveys, remote sensing, and citizen science contributions, each adhering to different protocols.
For researchers, implementing a standardized system could mean adopting existing guidelines or even creating new criteria that highlight best practices tailored to specific ecosystems. Ongoing training and workshops can raise awareness among scientists on the importance of data consistency, instilling a culture of quality from the ground up.
Interdisciplinary Communication
Ecological data science thrives on collaboration among various disciplines. However, each field has its own jargon, methodologies, and mental models, which can create barriers. An ecologist might have a different way of framing a problem than a data scientist. Strong interdisciplinary communication is therefore essential.
It’s important to foster environments where experts from different backgrounds feel comfortable sharing ideas and feedback. Consider hosting joint seminars or interdisciplinary meetings that emphasize common goals. Initiatives like this help build bridges, ensuring that findings are not just for a select audience but are accessible and comprehensible to all stakeholders involved in ecological matters.
Ethical Considerations in Data Use
The ethical dimension of data use cannot be overlooked. As data science progresses, the questions surrounding who owns the data, how it's collected, and how it is ultimately used become more prominent. This is especially true in ecology, where data often involves sensitive information regarding species conservation or indigenous lands.
Research practices should prioritize transparency and seek consent when applicable, especially in areas involving local communities. It’s imperative that data scientists consider the implications their research could have. Taking the time to develop guidelines for responsible data sharing not only protects vulnerable ecosystems but also strengthens public trust in research findings.
In summary, addressing the challenges in ecological data science not only enhances research credibility but also helps pave the way for actionable insights that can benefit conservation efforts worldwide.
Future Trends in Ecological Data Science
The realm of ecological data science is advancing at a rapid pace, reflecting the increasing complexity of environmental problems and the corresponding need for sophisticated analytical approaches. This section explores key future trends that will shape the field, focusing on advancements in technology, collaborative research, and integration into policy and management. Understanding these trends is essential for anyone involved in ecology, as they not only provide direction for future research but also underscore the importance of interdisciplinary approaches in solving ecological dilemmas.
Advancements in Analytical Technologies
The surge in computational power coupled with innovative software developments is transforming how ecological data is analyzed. Machine learning algorithms, for instance, have gained notable traction. They allow for the processing of vast datasets which were previously deemed too cumbersome to analyze. These technologies enhance predictive modeling, improving outcomes in biodiversity assessments and habitat preservation strategies. Notable advancements include:
- Big Data Processing: The ability to process and analyze large volumes of data, which enables ecologists to derive insights from complex ecological interactions.
- Real-time Analytics: With technologies like IoT (Internet of Things), data can be collected and analyzed in real-time, allowing for immediate responsiveness to ecological changes.
- Robust Statistical Methods: Enhanced statistical techniques enable more accurate inferences about populations from sampled data, pushing research methodologies into new territories.
"New technologies are not just tools, but gateways to previously unimagined patterns in ecological data."
These advancements are crucial in addressing the intricate layers of climate change, species extinction, and habitat fragmentation. However, researchers must remain vigilant about the consequences of these rapidly evolving tools, particularly in ensuring their accessibility and usability across various ecological disciplines.
Collaborative Research Initiatives
In ecological data science, the notion of collaboration is taking center stage. Academic institutions, NGOs, and governmental agencies are increasingly working together in interdisciplinary teams to tackle environmental challenges. Collaborative initiatives promote:
- Sharing of Data and Resources: Different stakeholders can pool their datasets, which enriches the research landscape and highlights patterns that might be missed in isolated studies.
- Diverse Perspectives: Bringing together experts from different fields allows for a more comprehensive understanding of ecological issues. For example, biologists, computer scientists, and sociologists can co-develop solutions that take into account biological metrics alongside human impacts on ecosystems.
- Capacity Building: Collaborative efforts often include training programs which help upskill participants in new analytical methods, ensuring that innovations are effectively disseminated among stakeholders.
As these partnerships strengthen, they provide a robust framework for responding to global environmental crises with an integrated approach that combines scientific expertise with practical, on-the-ground knowledge.
Integration with Policy and Management
The integration of ecological data science within policy and management frameworks is vital for facilitating evidence-based decision making. This alignment ensures scientific findings translate into actionable strategies to conserve ecosystems. Considerations include:
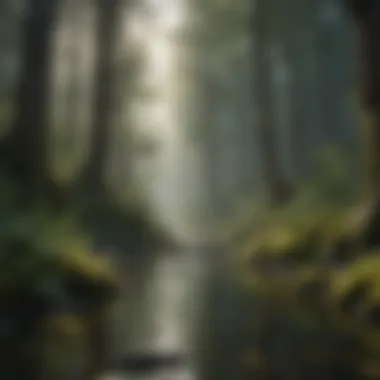
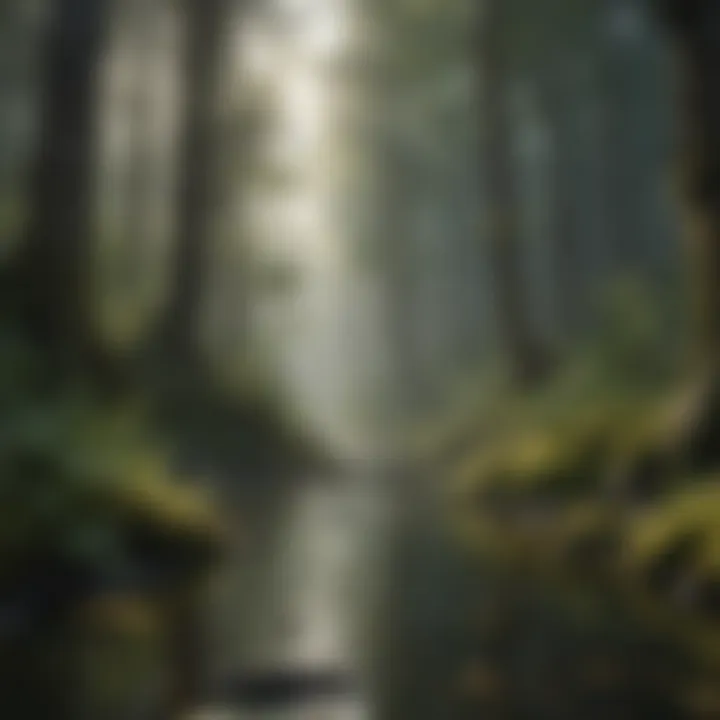
- Data-Driven Policy: Policymakers increasingly rely on quantitative data from ecological studies to make informed choices regarding land use, conservation priorities, and regulations. This trend aligns ecological insights with economic and social outcomes, fostering more sustainable practices.
- Adaptive Management: A flexible management approach allows for ongoing learning and adjustments based on new data. By utilizing real-time data acquirement through advancements in technology, managers can adapt strategies promptly to changing conditions.
- Public Engagement: Engaging communities in data collection and conservation efforts creates a sense of ownership and empowerment among stakeholders. This inclusivity bolsters public support and enhances the success of ecological initiatives.
Addressing ecological challenges requires a pragmatic approach that recognizes the interplay between scientific data and policymaking. As data science methodologies continue to evolve, their integration into policy will become increasingly vital in crafting sound environmental strategies that impact both conservation and human well-being.
Educational Pathways in Ecological Data Science
In the burgeoning field of ecological data science, the routes for education and training are not just peripheral; they're core to advancing the discipline itself. As the symbiosis between ecology and data analytics grows, so too does the demand for professionals who are well-versed in both realms. Educational pathways must therefore be thoughtfully designed to equip students with the skills necessary to navigate complex ecological systems and apply advanced data analysis techniques effectively.
The significance of these educational pathways cannot be overstated. They serve not only to create knowledgeable individuals but also foster a community that can collaboratively address pressing environmental issues. The complexity of ecological data—often characterized by vast amounts of information and intricate interdependencies—demands a well-rounded educational approach. Thus, prospective ecologists and data scientists alike must pursue academic programs that blend theoretical understanding with practical application.
Degree Programs and Courses
Degree programs specifically focused on ecological data science are paving the way for future experts in this integrative field. Many universities are now offering specialized programs that combine traditional ecology education with data science skills. These programs often include:
- Bachelor’s Degrees: A foundation typically in biological sciences, environmental science, or statistics—paired with electives in data management and programming.
- Master’s Programs: Advanced studies that dive deeper into research methodology, data visualization, and modeling techniques tailored for ecological data. These programs often culminate in substantial capstone projects that enable students to apply their learning in real-world contexts.
- PhD Programs: Focused research tracks that allow for in-depth exploration of particular ecological phenomena through rigorous data analysis, ultimately driving innovative research outcomes.
Key courses within these degree programs may encompass:
- Statistics for Ecology
- Geographic Information Systems (GIS)
- Ecological Modeling
- Environmental Data Science
- Remote Sensing and Analysis
By weaving together coursework in ecology with training in data science, these programs prepare students to think critically and work effectively at the interface of both domains.
Professional Development Opportunities
For those already in the field or recently graduated, professional development becomes a continuously evolving necessity. Engaging in ongoing learning ensures that practitioners remain adept at using the latest technologies and methodologies. Here are some valuable ways to pursue professional development in ecological data science:
- Workshops and Short Courses: Various organizations and institutions run workshops focused on practical skills, such as programming in R or Python specifically for ecological data analysis.
- Conferences and Symposiums: These events are goldmines for networking and knowledge sharing, often spotlighting recent advances and case studies in ecological data science.
- Online Certifications: Programs from platforms such as Coursera and edX can be particularly useful, offering flexible options for professionals to gain new competencies in relevant areas.
- Mentorship Programs: Engaging with a mentor who possesses experience in ecological data science can provide personal guidance and insights into career navigation.
Investing in professional development is crucial in a dynamic field such as ecological data science; it helps ensure that knowledge and skills remain relevant and cutting edge.
The combination of robust educational pathways and continual professional development equips individuals to contribute meaningfully to ecology and environmental conservation. The interplay of skills garnered through degree programs and further refined through ongoing learning creates a workforce ready to tackle the multifaceted challenges our planet faces today.
Case Studies in Ecological Data Science
Case studies serve as vital touchstones in the field of ecological data science, providing concrete examples of how theory translates into practice. They illustrate the intricacies of applying data analysis to real-world ecological challenges, showcasing a spectrum of techniques and approaches that yield actionable insights. By examining these successful projects, students, researchers, educators, and professionals can glean strategies applicable to their own work. Moreover, these case studies often highlight the collaborative nature of ecological research, bridging gaps between disciplines and fostering innovation.
Successful Conservation Projects
Successful conservation efforts often hinge upon the insights drawn from ecological data science. One significant example is the recovery strategies for the California condor. This majestic bird, once on the brink of extinction, has seen a gradual population increase due to meticulous data collection and analysis. Researchers employed GPS tracking and habitat modeling to understand the condor's movement patterns and habitat requirements. This data was crucial in making informed decisions regarding breeding programs and habitat restoration initiatives. The blend of field data and analytical skill has transformed conservation strategies, demonstrating their potential to safeguard biodiversity.
Furthermore, a noteworthy project involved the rehabilitation of coral reefs in Australia. Researchers utilized underwater drones equipped with advanced imaging technologies to monitor reef health and assess the impact of climate change. By integrating this data with local ecological knowledge, they implemented targeted restoration efforts, enhancing the resilience of these fragile ecosystems. This case underscores how technology paired with traditional ecological practices can yield transformative results in conservation.
Innovative Use of Technology in Research
In the realm of ecological research, technology plays an indispensable role in enhancing our understanding of environmental systems. A standout example is the deployment of big data analytics in tracking migratory patterns of wildlife. The African elephant, for instance, has been the focus of numerous studies which utilize satellite imagery and machine learning algorithms. These technologies enable researchers to parse through vast datasets, identifying migration corridors and human-wildlife conflict zones with unprecedented precision.
Another innovative use of technology can be found in the realm of citizen science, particularly seen in platforms like iNaturalist. This approach allows everyday individuals to contribute to ecological data collection by submitting observations of flora and fauna. The integration of such data into scientific research enriches datasets, providing a broader perspective on biodiversity and ecosystem health. The analysis of this crowd-sourced information can lead to significant findings that would be otherwise unattainable.
"The combination of grassroots observation and advanced analytical methods holds the key to enriching our understanding of ecosystems. It invites everyone to be a part of ecological research, making science more inclusive and dynamic."
In summary, case studies in ecological data science are crucial for demonstrating how analytical tools can address real-world ecological challenges. By learning from successful conservation projects and innovative uses of technology, stakeholders in the ecological field can enhance their approach and develop more effective strategies for preserving our natural world.
Concluding Thoughts
The field of ecological data science acts as a bridge between natural ecosystems and the data-driven world, revealing how meticulous analysis can enhance our understanding and management of ecological systems. In this article, we've traveled through numerous dimensions of this growing discipline, reflecting on its significance in today’s environmental landscape.
The Importance of an Interdisciplinary Approach
In ecological data science, the need for an interdisciplinary approach can’t be overstated. Ecology itself is an inherently multifaceted subject, encompassing aspects of biology, geography, and environmental science. When combined with data analysis, it invites disciplines like computer science, statistics, and social sciences to join the conversation.
- Cultivating Collaboration: Collaborative projects often lead to innovative solutions that individual disciplines may overlook. For instance, ornithologists partnering with data scientists can analyze avian migration patterns far more comprehensively through machine learning models than by traditional methods. This cooperative spirit is essential as real-world problems don’t fit neatly into academic silos.
- Broadening Perspectives: Bringing diverse perspectives together can also lead to refined questions and more robust methodologies. A background in behavioral science can inform how certain ecological data might be interpreted, enhancing the depth of analysis.
By encouraging interdisciplinary efforts within ecological data science, we bolster our collective ability to tackle complex environmental issues in a more holistic manner.
Encouraging Future Research Directions
The roadmap ahead for ecological data science is filled with potential. As certain trends develop in technology and methodology, it's vital to sustain momentum in research. Here are a few directions worth considering:
- Integration of AI: The future will likely see increased integration of artificial intelligence in managing and analyzing ecological data. Sophisticated models could enhance predictive capabilities, making assessments on climate scenarios more accurate.
- Real-Time Data Utilization: Advances in sensor technologies and satellite imagery present fresh opportunities. Real-time data collection will provide ecologists with immediate insights into changes in ecosystems, allowing for faster response strategies.
- Public Engagement: Future studies should also focus on expanding public involvement in data gathering. Citizen science initiatives can amplify data collection efforts, encouraging broader community participation while enriching the dataset available for analysis.
Indeed, the fusion of these elements can stimulate comprehensive research that is applicable not only in the lab but also in practical conservation efforts.
"To safeguard our planet's future, melding ecological insight with data science remains not only beneficial but crucial."
As we reflect on the knowledge imparted in this article, the interplay between ecology and data science emerges as a profound narrative. Maintained curiosity, interdisciplinary engagement, and adaptive research methodologies will be pivotal in addressing the ecological challenges we face today. Only then can we evolve into proficient stewards of the environment.